Companies have high hopes for transformational technologies, especially those that leverage the vast amount of data being collected. Approaches using methods such as artificial intelligence (AI), machine learning (ML) and deep learning are becoming increasingly accessible and gaining significant traction, as they allow for deep insights to be extracted from large and varied data sets. As noted in the 2019 IT Audit survey by Protiviti and ISACA, this transformation has the potential to fuel long-term growth.
However, as Protiviti’s recent global AI survey reveals, most companies are still at the starting gate when it comes to figuring out the answers to basic questions: What are the possible use cases for AI? Can we measure ROI? What data do we have and how usable is it? Do we have the internal and/or external resources required for good data management and governance, and the necessary analytics capabilities? That holds true within the internal audit function as well, as audit leaders look for ways to both put these technologies to use in their departments as well as evaluate the risk of adoption and use within their organizations.
These topics are front of mind in next-gen audit discussions with our clients and we want to share some of our own insights to illustrate what is possible and how to go about setting priorities and moving forward with a substantive and effective transformation. AI can seem like an out-of-reach concept reserved only for those with advanced degrees and background in statistical mathematics, but that is a misconception when it comes to practical applications. In this blog we shed light on the basic elements of AI and provide some thoughts on how internal audit organizations can get started in their evaluation and use of AI methods and technologies, along with some of the ways internal audit functions are already putting those elements to use.
AI Basics
It might be helpful to begin with some definitions. Artificial intelligence is an umbrella term for several different technologies that allow computer systems to perform tasks that normally require human intelligence, such as visual perception, speech recognition and decision-making. The following are subsets of AI:
- Machine Learning (ML) is the use of statistical techniques to enable computers to “learn” with data without being explicitly programmed. ML can detect patterns or apply known rules to predict outcomes, detect anomalies and yield insights.
- Deep Learning (DL) is a subset of ML that attempts to model the way connections work in the human brain by applying successive layers of representations to teach computers to learn by example and make decisions themselves.
- Natural Language Processing (NLP) is the use of computational linguistics and artificial intelligence to enable computers to understand and interpret human language, including speech and the written word.
- Computer Vision is the ability of machines to recognize and draw data/information from images such as pictures and videos.
The following diagram illustrates how all of these subsets relate to AI and to each other, as well as to robotic process automation (RPA) — a popular technology solution that imitates human actions as opposed to human thinking:
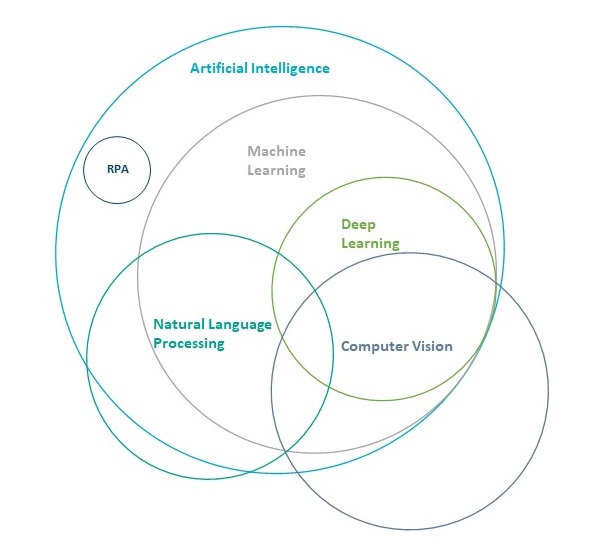
Application to Internal Audit
As applied to internal auditing, ML can help auditors identify patterns and trends from large data sets (that might otherwise be hard or overly time consuming to identify) and provide insight to support risk assessment, project scoping, sub-population identification, issue identification, quantification, and more. Moreover, achieving this outcome does not require a deep level of technical capability. Internal audit teams can apply, with limited configuration, “off-the-shelf” algorithms such as k-means clustering, decision tree-based models and affinity analysis to identify items within a population that have a statistically meaningful similarity between them and, conversely, identify anomalies, or outliers, that don’t follow the rules and therefore warrant closer study.
Some internal audit teams have already begun to apply ML to various fraud-prone or high-risk areas such as purchasing, manual journal entries, travel and entertainment, and system activity. These tools are proving to be helpful not only to internal auditors but also to their business partners who are able to use them to detect and correct (and even predict) anomalies before they can escalate into actual incidents.
NLP is being be used to scan through large volumes of documents such as contracts, loan records and other unstructured data sources for critical information. This can then be combined with other AI components (such as ML, DL and RPA) to classify documents according to type, extract relevant information and perform analysis.
This ability to work with large quantities of unstructured data, classify documents and content, and identify and extract relevant data points is a critical advancement over traditional methodologies in which data fields had to be clearly defined and automated processing was limited to only the most structured and trusted data sets.
Facing the Challenges
Sound data management and governance represent the most significant and legitimate barrier to AI adoption for most organizations. With the rapid increase in the capture, processing and storage of new data, organizations need to take a hard look at how they are organizing themselves (from people, process and technology perspectives) to ensure that data is properly organized and accessible, has integrity and is appropriately secured, allowing it to be used as an enterprise-value enabler. Internal audit can clearly help here by taking a robust look at an organization’s data management practices and capabilities – a topic that warrants its own separate discussion.
Before internal auditors can implement artificial intelligence tools, there are four basic questions that must be answered in the affirmative. Those questions are:
- Do we know where our data is?
- Is the “version of the truth” known and agreed upon?
- Is the data accessible?
- Is the data reliable (i.e., is data integrity assured)?
To date, most of internal audit’s emphasis on AI has come from the perspective of how to effectively govern and review the technology, but as we pointed out here, there is also a significant opportunity to use these tools throughout the internal audit lifecycle. In addition to some of the “off-the-shelf” methods mentioned earlier in this blog, there is a growing number of packaged solutions, with AI components, available in the market and moving from emerging to established. All of these available tools and products lower the barrier to AI adoption. Of course, more advanced and customized solutions will require commensurately advanced skills but internal audit should not shy away from AI because they may lack Ph.D.-level statisticians on their team.
As internal audit continues to evolve into a next-gen function, the role of AI in audit should be clearly defined in strategic plans, and internal auditors should collaborate actively with other departments and third parties, including data scientists, business partners and IT to ensure that the internal audit team has access to the infrastructure, technology and expertise required to apply AI in the most appropriate areas. The goal is to help internal audit realize the value potential related to efficiency, effectiveness, improved coverage and internal audit team member engagement and satisfaction. This collaborative and strategic approach to AI will also help propel internal audit to the innovative edge of the organization, positioning it as a high-value, trusted advisor and making the audit function an exciting and cutting-edge place to work. At Protiviti, we strongly believe that being an internal auditor has never been more exciting.
Next-gen audit, data analytics and increased productivity through intelligent automation are ongoing themes here on The Protiviti View. Subscribe to follow the discussion. Also, learn more about Protiviti’s global consulting services.
Madhumita Bhattacharyya, Managing Director and Practice Leader for Business Strategy and Advanced Analytics at Protiviti, contributed to this content.
Add comment